Article
Data Warehouse Development: A Guide and Case Study
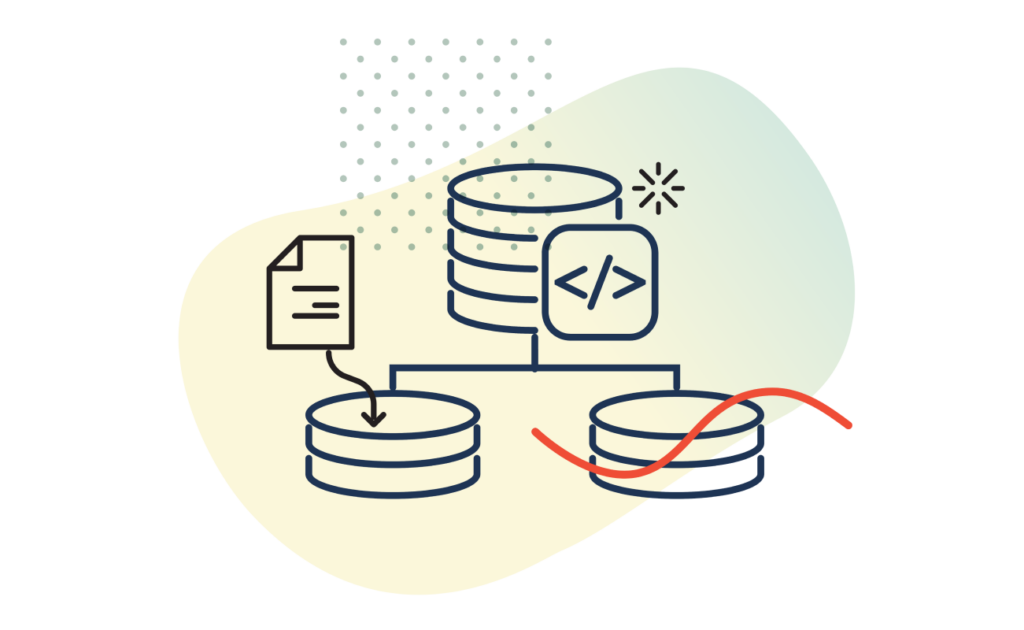
To begin this post about the ins and outs of data warehouse development, I’d like to acknowledge the role Brian Ellis and Steve Hulet played in helping me refine the content. Are you interested in how Fresh can help you with your data warehousing needs? Would you like to connect just to chat about the discipline? Look no further than Brian and Steve, two exceptionally smart and highly skilled software engineering thought leaders who’ve made Fresh’s software development wing what it is today: a principle-driven, client-centric team that provides best-in-class software solutions for clients and partners in every major industry.
And now, for the post! What is data warehouse development and how does it benefit businesses?
Making data-driven decisions with confidence
Modern businesses are awash in data about their people, processes, and technology. To triangulate between disparate data sources, well-organized data management systems are not just beneficial—they’re essential.
Enter data warehouse development, a strategy focused on creating systems to store and manage large volumes of data from various sources.
In its Voice of the Enterprise Survey, S&P Global reported that, in a survey of organizations regarding whether their strategic decisions are data-driven, ~25% said almost all decisions are data-driven. In the same survey, 44% of respondents attested that most decisions are data-driven. Yet, according to a survey conducted by Precisely, “76% [of survey respondents] say data-driven decision-making is their #1 goal for data programs, yet 67% don’t completely trust their data.”
Research indicates widespread consensus that data-driven decision-making is essential. But there’s also a notable distrust in the data itself.
Taking these factors into consideration, modern organizations must build systems that:
- Enable data-driven decision-making at scale across their organization
- Provide clean, reliable data pipelines between disparate systems
- Foster trust that the data is good and that any subsequent decision-making will be based on trustworthy data
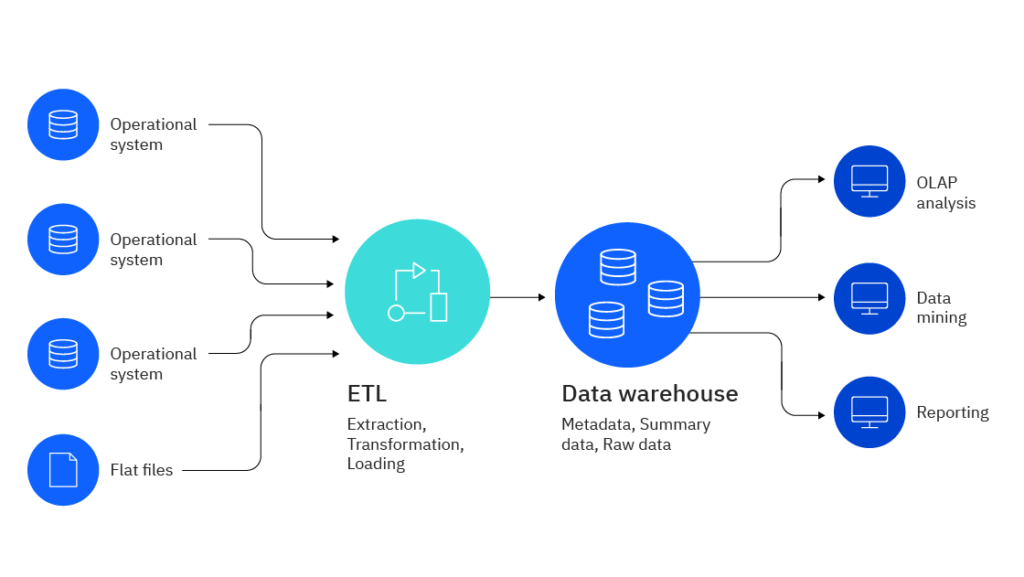
So, what is a data warehouse exactly?
At the most basic level, a data warehouse is a centralized repository to store large volumes of data. Data warehouses serve as the foundation for the Business Intelligence (BI) systems organizations use to carry out their vital functions: performing complex data analyses, generating insightful reports, making informed business decisions, and much more.
Several core components of a data warehouse include:
- Data sources (depending on the size of the data warehouse, there can be few sources or many)
- ELT (Extract, Load, Transform) or ETL (Extract, Transform, Load) tools
- A centralized database (the repository)
- Integrated BI tools and data modeling functionality
- Rigorous documentation of organizational best practices and standards for data governance
Organizations that spend time defining these core components are better able to maintain data quality, security, and governance. This is a critical exercise for undertaking truly impactful digital transformation initiatives related to the use of data.
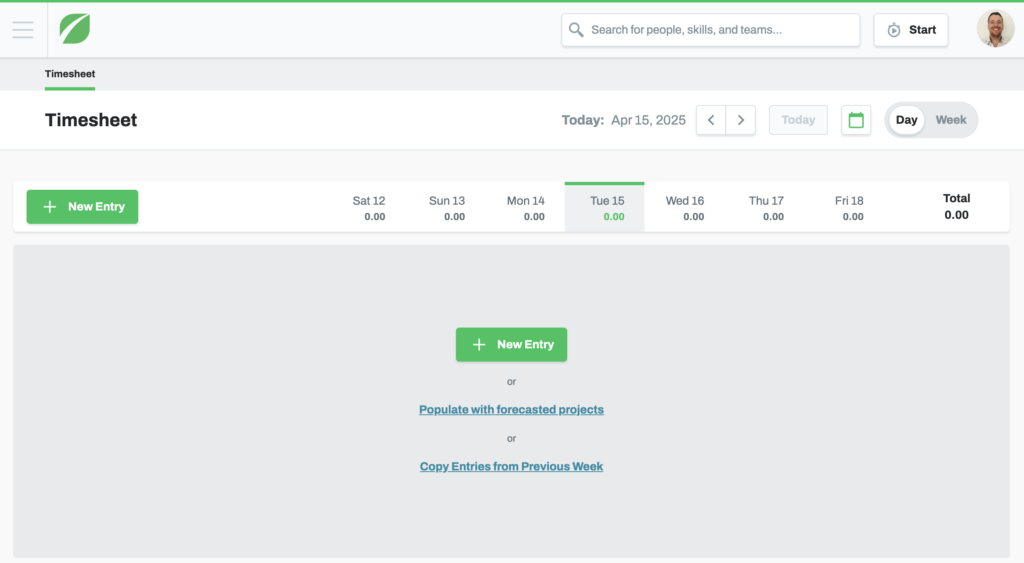
A financial data warehouse: Fresh’s initial use case
Approaching the challenge of organizing and leveraging data more efficiently at Fresh, our software development engineers sought to address a financial data management and reporting challenge.
The goal? Building a robust data warehouse system to streamline our internal processes.
The project showcased an example of the transformative power of data warehouse development and the value it holds as organizations seek to get the most out of their data. Leveraging this foundational work, our team is equipped to extend that value proposition to our clients and partners.
In my interview with Brian Ellis, a full-stack developer at Fresh who played a critical role in the data warehouse project, he discussed what he sees as the fundamental value of a data warehouse:
“With access to a data warehouse, you have data and statistics to behind all of your decisions. A data warehouse helps us make decisions that are data-driven, rather than being based on what we’re feeling. With our internal data warehouse project—gathering project data from various sources for financial reporting—PMs can say, I know this project is performing or underperforming, versus I think. Ultimately, a data warehouse gives decisions more weight and more information to make informed decisions.”
It’s easy to imagine the value this would hold for other organizations. A wholesale food distributor with performance data related to the products they offer and the ability to organize inventory accordingly. A construction materials delivery platform that leverages robust sensor data for route planning and real-time analytics.
The possibilities are limitless.
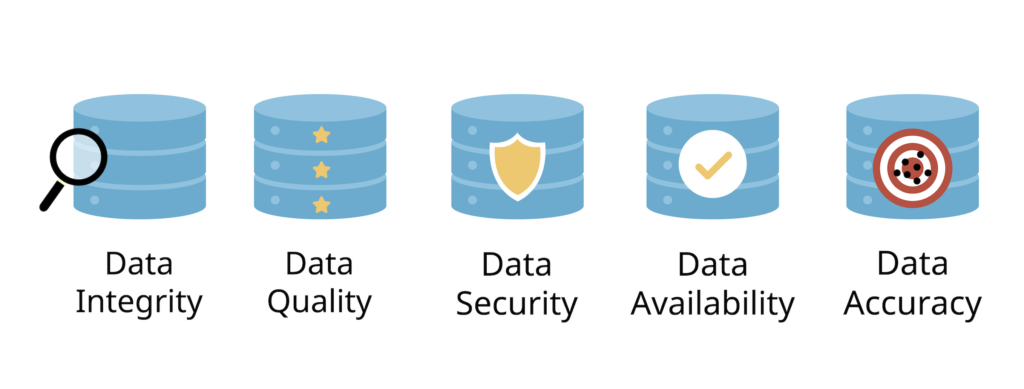
8 steps to building a data warehouse—How we did it at Fresh
Developing a data warehouse is a multi-faceted process involving several critical steps. The steps below are one possible approach; however, they’re not the only option for developing a scalable and efficient data warehouse. Each organization should consider its unique use case and adopt a process for data warehouse development that supports its core objectives and the capabilities of its team.
When I asked Brian for his thoughts on the value of having a process to guide the development team, he spoke to the importance of consistency:
“A process allows you to define requirements, do technical research, build a test version, and conduct the testing itself. Earlier in my career on other software development projects, the test version sometimes became the production version, while being based on assumptions. Then, if it’s broken, it’s broken. Even if it feels like it’s inefficient to follow a process, pulling things back and having structure on the front end of a project makes it easier to maintain quality in the long run.”
As your team sets out to build its data warehouse, consider similar steps to accelerate your path to building a high-quality end product.
Step #1: Define Business Requirements
Task: Gather a list of business needs. Focus on strategic decision-making goals and other critical objectives.
Objective: The first step involves aligning the data warehouse with an organization’s larger Business Intelligence (BI) strategy. A retail company might need access to real-time sales data analysis across regions. A logistics organization might face challenges around storing data across systems. Challenges regarding operational efficiency are somewhat common in organizations, regardless of their industry.
The Fresh Use Case: Defining Business Requirements
At Fresh, managing client billing requires the timely movement of data between systems. The decision to build a new data warehouse stemmed from the need for efficient data management.
Our core goal was simple: we set out to create a data warehousing solution that ensured financial data could be moved between different systems used by the finance department. Our primary business requirement was making it easier for our finance team to handle billing and time management. Additionally, we sought to cut manual intervention, a time-consuming and resource-intensive process.
Step #2: Analyze Source Data
Task: Identify data sources and outline systems of record, prioritizing data integration capabilities.
Objective: This step involves assessing the quality and structure of data from various sources. Examples include CRM systems, ERP platforms, and external databases. Data integration techniques are essential to ensure seamless connectivity between data sources.
The Fresh Use Case: Analyzing Source Data
Fresh Teams, our proprietary time-tracking application, was one repository of source data we sought to transfer into our accounting software, although we have numerous other sources of data as a part of our accounting workflow.
Step #3: Develop the Concept and Select the Ideal Platform
Task: Define features. Choose deployment options (cloud, on-premises, hybrid). Select the technologies best suited for data modeling and data analytics needs.
Objective: This step involves evaluating different Enterprise Data Warehouse (EDW) solutions. Considerations include scalability, performance, and compatibility with your organization’s existing systems. A company might choose a cloud-based EDW based on its need for flexibility and cost-effectiveness,
The Fresh Use Case: Developing the Concept and Selecting the Technology Platform
We chose a cloud-based data warehouse with GCP BigQuery. Then, thinking about the data pipelines and integrations within the data warehouse, the decision came down to Meltano and, eventually, Astronomer.
Initially, for data pipelines within the data warehouse, we chose Meltano Cloud for its plug-and-play capabilities. Meltano allowed us to integrate various components with speed. This enabled our teams to configure the data system with ease. However, as Meltano Cloud shut down, we were forced to look for alternative solutions.
Shifting away from hosting on Meltano Cloud—although we still use Meltano as a part of our data pipelines—we eventually transitioned to Astronomer, a vendor specializing in managing Airflow projects. This transition was a strategic decision to improve our data handling capabilities. Using Astronomer brought several benefits. These included superior management of Airflow jobs and streamlining the Meltano project integration.
Step #4: Project Roadmap Development
Task: Align project milestones with business objectives. Examples include robust performance monitoring, efficient metadata management, etc.
Objective: This step involves creating a detailed timeline for implementing data warehouse components. Components include ETL (Extract, Transform, Load) processes, data marts, and other key functionality. It’s essential to consider your organization’s data governance policies as well.
The Fresh Use Case: Astronomer’s Fit with our Project Development Roadmap
The evaluation of our options involved several decision-making criteria. Fresh management’s preferences leaned toward solutions that provided robust performance while remaining cost-effective. Astronomer offered a compelling option given its capacity to host complex data projects.
We also compared Astronomer with AWS. Astronomer offered competitive pricing alongside tailored support for our workflow. Additionally, Astronomer’s expertise in running data pipelines through Airflow allowed us to focus on innovation rather than the underlying infrastructure.
Platform comparison, selection, and implementation schedule are critical considerations in the roadmapping phase.
Step #5: Design the Solution
Task: Define data cleansing and security policies, data models, and data warehouse architecture.
Objective: This step aims to build a robust data security framework. Goals include protecting sensitive information and designing efficient data models for complex analytics. For example, a bank exploring data warehouse solutions might install role-based access control and encryption to protect financial data.
The Fresh Use Case: Designing the Ideal Solution
Any failure to address our data management issues had significant implications.
We set out to build a data warehouse solution because manual data processes burdened our finance team. These inefficiencies affected the team’s productivity and diverted attention from essential work. But without a reliable data pipeline, our finance team would perform manual data entry. The risk was not only wasting time but also increasing the risk of manual errors.
Another risk to consider was security, given that we were dealing with financial data. Automating our data pipelines was crucial. In parallel to productive efforts, security, data cleansing, and system architecture were critical.
Step #6: Customize Platform & Develop ETL/ELT Pipelines
Task: Deploy the platform. Ensure robust integration processes and appropriate data integration pipelines, for data velocity and data completeness. Keep in mind that ELT (Extract, Load, Transform) and ETL (Extract, Transform, Load) are both forms of data pipelines, but aren’t interchangeable. ELT is a data integration approach where data is first extracted and loaded into a destination system in a raw format, then transformed within that system. ETL extracts data from one or more sources, transforms it into a usable format, and loads it into a target database or data warehouse. Being cognizant of the differences and what is appropriate for your system is critical.
Objective: This step involves two focuses. The first is configuring your selected EDW platform. The second is creating the appropriate data pipeline workflows to extract source data and load it into the data warehouse.
The Fresh Use Case: Customizing the Platform via ETL/ELT Pipelines
Understanding the significance of data science and its applications was vital. We leveraged data warehouse development principles and implemented ELT (Extract, Load, Transform) pipelines to facilitate data processes.
Utilizing Apache Airflow and Docker enabled us to enhance data workflow execution. Docker’s containerization enabled us to quickly develop and deploy custom solutions.
Step #7: Migrate Data
Task: Transfer data into the warehouse, ensuring data quality and smooth data staging.
Objective: This step involves data cleansing, validation, and building a data staging layer. There are often different sections of a data warehouse—one for the raw source data, another for the transformed “cleansed” data, etc. It’s crucial to maintain data consistency and accuracy during this phase.
The Fresh Use Case: Migrating Vital Data
Taking the steps for data transfer has already demonstrated significant ROI. We estimate that our finance team’s workload has been reduced by multiple hours monthly. This enables them to put more time toward strategic planning and client support. Furthermore, the financial benefits extend beyond time savings. Automation minimizes manual errors, reducing the need for adjustments in scope or estimation.
Step #8: Monitor and Optimize
Task: Fine-tune performance through performance monitoring and adjustments. Ensure the warehouse meets business analytics requirements.
Objective: This step involves performance analysis, query optimization, and implementing automated data management. An example of ongoing optimization could involve leveraging OLAP Cubes for improved query performance.
The Fresh Use Case: Monitoring, Optimization, and Future Iteration
Looking ahead, our vision is to establish an automated financial environment. Anna Kuznetsov, a Senior Accountant on our finance team, often had to manually check transactions to ensure accuracy. Via process automation, we enabled her to perform critical tasks without manual intervention.
The new system has underscored the importance of standardization, too. Standardization is critical as we monitor, optimize, and iterate on the data warehouse. By utilizing consistent frameworks and clear documentation, such as tailored Read.me files, we’ll ensure teams have the resources they need.
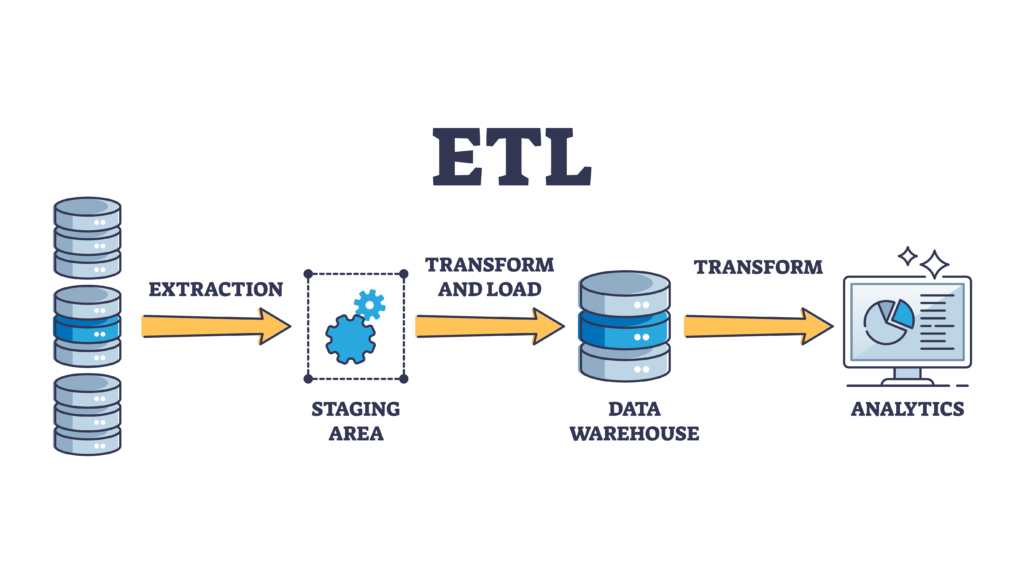
Additional Industry Use Cases for Data Warehouse Development
The ongoing transition to more automated financial workflows simplifies Fresh’s internal operations. It also serves as a case study for process improvement via data warehouse development. Organizations across industries can adopt similar practices to boost productivity.
During my conversation with Brian, I was curious about what the alternative to having a data warehouse would be. What risk would not having a data warehouse carry for an organization?
Brian spoke about the virtue of having “all your information in one place”:
“Without a data warehouse in our situation, we’d have a bunch of different systems that we go to to find information; critical project information spread across four or five different applications. In order for our financial team to find the project information they’d need, they’d have to go to each of the systems separately; a ton of manual work. A data warehouse makes things more consistent and straightforward, simplifying where data passes through and the systems it goes into.”
Consistent, straightforward, and simplified data pieplines—it’s a compelling value proposition. Let’s talk about what that could look like in various industries.
Business Intelligence and Data Analytics (Multi-Industry Application)
Business Intelligence (BI) and data analytics are broad categories. They’re also the most clear-cut examples of why data warehouse development is valuable. Enabling rapid data-driven decision-making is critical for modern businesses across the spectrum.
Example: A manufacturing company might use BI tools to analyze production data from multiple plants. By identifying bottlenecks and optimizing processes, seamless multi-plant integration is well-within reach.
Marketing & Sales (Multi-Industry Application)
Marketers and sales teams can use data warehouse tools to analyze trends and optimize campaigns. Integrating data sources in MarTech and business development enables better alignment. Integrated data is one of the most crucial challenges to solve in RevOps models—sales, marketing, and business development departments must have access to the same centralized, accurate repository of data as they make their critical decisions.
Example: Data warehouses enable B2B marketers to segment customers based on historical data. Segmentation enables personalized marketing messages and effective measurement across marketing channels. Sharing the data with systems used by sales increases visibility, transparency, and confidence.
A B2C example is Netflix. B2C companies strive to create a better experience for users is critical. Marketing the Netflix value proposition is centrally important. Netflix’s data warehouse approach handles the “petabytes of data” their platform processes. Efficiency is critical for making the platform affordable and highly performant from the standpoint of giving users access to the shows and movies they love.
Financial Analysis
Centralizing financial data is essential to enhancing profit maximization strategies and securities compliance. These are two of the many use cases for financial organizations flooded with sensitive financial data.
Example: Financial institutions can leverage data warehouses to consolidate data from various sources. This enables comprehensive risk assessments and regulatory compliance reporting. In 2023, for example, J.P. Morgan launched its Securities Services Data Mesh. The platform “enables investors to retrieve critical investment data held by J.P. Morgan’s Custody, Fund Accounting and Middle Office services, using cloud-native channels including REST APIs, Jupyter notebooks and the Snowflake Financial Services Data Cloud.”
Retail Operations
Retail companies and wholesale distributors benefit from centralized data access. Leveraging data warehouses and related technology enhances inventory management, merchandising, and CRM performance.
Example: Retailers can use data warehouses for real-time analytics. This enables customer behavior analysis, optimized product placement, and dynamic pricing strategies.
Walmart uses ETL tools to process data across its massive global supply chain. “With more than 245 million customers visiting 10,900 stores and with 10 active websites across the globe,” centralized sales and product data is critical.
Banking & Risk Management
Banks can leverage data warehouse strategies for market trend analysis, policy evaluation, and risk management. For resource-limited regional banks and credit unions, strategic data use is critical. Bigger banks have more expansive resources. That said, cultivating better data and leveraging it for decision-making is an edge any institution can achieve.
Example: Banks can utilize data warehouses to consolidate customer data, transaction histories, and market information. Outcomes include enabling more accurate credit risk assessments and fraud detection. Data management is central to invisible banking, open banking, and smart branch strategy.
Government Services
Government use cases for data warehouses include managing compliance, payroll, and recruitment processes. Government agencies can also use data warehouses to integrate data from disparate departments.
Example: Government agencies can use data warehouses to integrate data from various departments. In so doing, they can improve service delivery and policy-making through data-driven insights. Government organizations provide open data hubs that can be leveraged by private-sector companies. The ability to share classified data inter-departmentally is also critical.
Hospitals and Healthcare
Data warehouses integrate diverse healthcare data, enabling comprehensive patient insights and data-driven decision making.
Example: A hospital might use its Enterprise Data Warehouse (EDW) to analyze data, identify patterns, and determine probable treatment outcomes. This supports improved clinical protocols and enhanced patient care through secure data analytics. An industry example includes the UC Health Data Warehouse (UCHDW). The UC Health System built “a secure central data warehouse for operational improvement, promotion of quality patient care, and to enable the next generation of clinical research” for its “18 health professional schools, six medical centers, and 10 hospitals.”
Logistics and Warehousing
Data warehouses in logistics centralize operational data. This enables efficient supply chain management, inventory optimization, and real-time tracking of goods.
Example: A global logistics company might use data warehouses to integrate distribution centers. With access to historical shipping patterns and current inventory levels, logistics companies can optimize route planning, improve demand forecasting, and enhance the operational efficiency of supply chains.
One example is SkyCell, a logistics provider for “deep-frozen medicine.” Pharmaceutical companies can leverage SkyCell’s deep-frozen containers to track and manage billions of data points about temperature, location, and time and pipe that data into their workflows.
Robotics and Automation
Data warehouses in robotics and automation integrate diverse sensor data. This enables advanced analytics, machine learning, and real-time decision-making for robotic systems.
Example: An agricultural robotics company might leverage data warehousing for robot sensors. Centralizing data supports MLOps for continuous model improvement, enhances robot perception algorithms and perception libraries, and optimizes fleet communication. Outcomes might include more efficient crop monitoring, precise harvesting, and adaptive autonomous navigation in dynamic field environments.
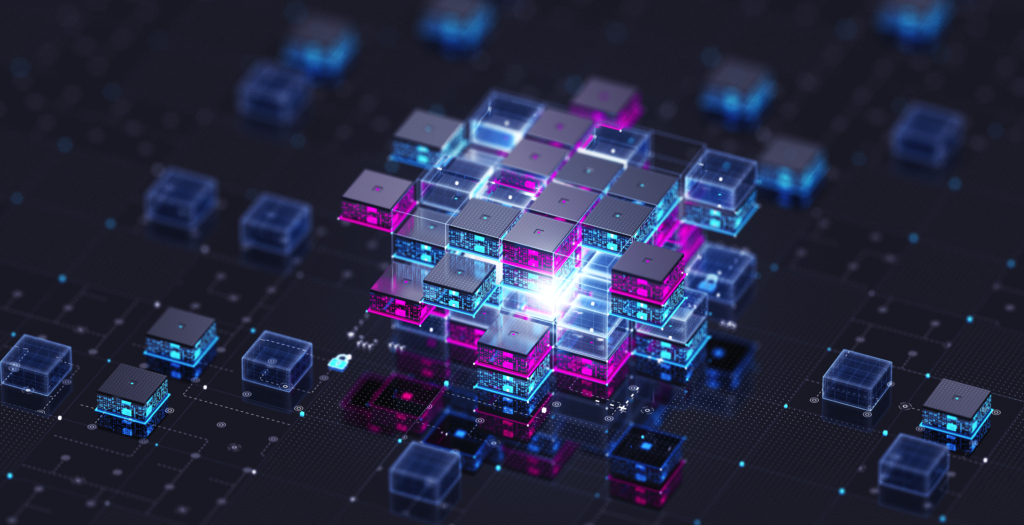
The transformative potential of data warehouse development
Fresh’s data warehouse development project positively impacted our financial operations. But the value of data warehouse development extends across industries.
As my conversation with Brian Ellis about the financial team project wrapped up, he spoke about how data warehouses provide a combination of benefits that ultimately make people’s lives easier:
“Data-driven decisions, the ability to automate manual processes—if I can save someone in finance an hour a week, that’s beneficial.”
Like they did for our financial department, Fresh software engineers’ data warehousing, automation, and technology consulting services can help streamline your processes as well.
Whether we help build the system or provide expertise on optimizing what you have in place, we’re here to help.